Identification of temporal patterns in the seismicity of Sumatra using Poisson Hidden Markov models
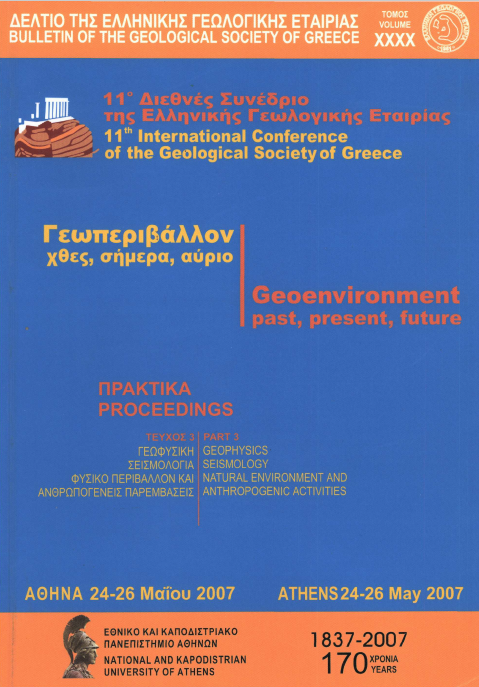
Abstract
On 26 December 2004 and 28 March 2005 occurred two of the largest earthquakes of the last 40 years between the Indo-Australian and the southeastern Eurasian plates, with moment magnitudes Mw=9.1 and Mw= 8.6 respectively. Complete data (mb > 4.2) of the post-1993 time interval (Fig. 1) have been used to apply Poisson Hidden Markov Models (PHMM in identifying temporal patterns in the time series of the two main shocks. Each time series consists of earthquake counts, in given and constant time units, in the regions determined by the aftershock zones of the two main shocks. In PHMM each count is generated by one of m Poisson processes, that are called states. The series of states is unobserved and is, in fact a Markov chain. The model incorporates a varying seismicity rate; it assigns a different rate to each state, and detects the changes of the rate over time. In PHMM, unobserved factors related to the local properties of the region, affect the earthquake occurrence rate. Estimation and interpretation of the unobserved sequence of states that underlie the data contribute to a better understanding of the geophysical processes that take place in the region. We applied PHMM to the time series of earthquakes preceding the two main shocks, and we estimated the unobserved sequences of states that underlie the data. The results showed that the region of the 26 December 2004 earthquake was in state of low seismicity during about 400 days before the earthquake occurrence. On the contrary, in the region of the 28 March 2005 earthquake a transition from a state of low seismicity to a state of high seismicity was observed immediately after the occurrence of the big earthquake of 26 December 2004.
Article Details
- How to Cite
-
Orfanogiannaki, K., Karlis, D., & Papadopoulos, G. A. (2007). Identification of temporal patterns in the seismicity of Sumatra using Poisson Hidden Markov models. Bulletin of the Geological Society of Greece, 40(3), 1199–1206. https://doi.org/10.12681/bgsg.16872
- Section
- Geophysics and Seismology

This work is licensed under a Creative Commons Attribution-NonCommercial 4.0 International License.
Authors who publish with this journal agree to the following terms:
Authors retain copyright and grant the journal right of first publication with the work simultaneously licensed under a Creative Commons Attribution Non-Commercial License that allows others to share the work with an acknowledgement of the work's authorship and initial publication in this journal.
Authors are able to enter into separate, additional contractual arrangements for the non-exclusive distribution of the journal's published version of the work (e.g. post it to an institutional repository or publish it in a book), with an acknowledgement of its initial publication in this journal. Authors are permitted and encouraged to post their work online (preferably in institutional repositories or on their website) prior to and during the submission process, as it can lead to productive exchanges, as well as earlier and greater citation of published work.