Ethical Issues Arising from the Use of AI in Drug Discovery
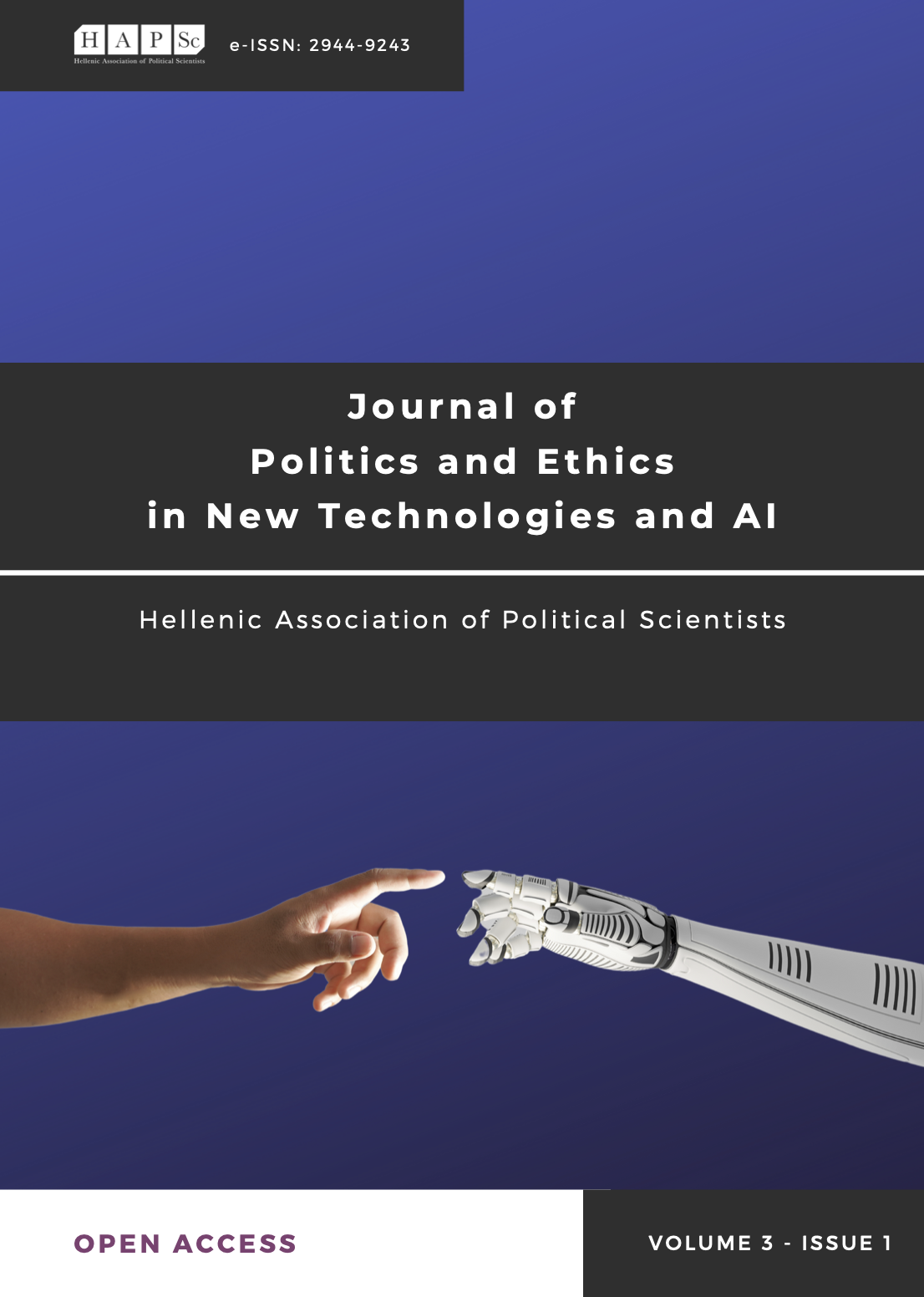
Abstract
Lately, the pharmaceutical industry has been implementing artificial intelligence (AI) in research in multiple ways for the purpose of drug discovery. AI has great benefits to offer by performing tasks that a human may not be capable of. However, it also raises many ethical issues that need to be resolved. After describing the general picture, this paper explores the benefits and disadvantages of AI and attempts to find answers to the challenges it poses in order to make sure that its use will be ethical, fair, unbiased and beneficial to people. The challenges addressed below focus on ensuring patients’ safety from biased AI algorithms and violation of their data privacy, the need to train and inform staff properly, as well as the necessity of achieving an optimal level of trust towards AI. In the end, some policy recommendations are being presented, aiming to contribute to the centralized approach and to the balanced sense among the uses of AI. The key to success is always taking into account human agency in order to make AI’s use transparent and robust, while minimizing its risks.
Article Details
- Section
- Commentaries

This work is licensed under a Creative Commons Attribution 4.0 International License.
Authors retain copyright and grant the journal right of first publication with the work simultaneously licensed under Creative Commons 4.0 (CC-BY 4.0) license, that allows others to share the work with an acknowledgement of the work's authorship and initial publication in this journal.