Utilizing Synthetic Data and Artificial Neural Networks for Clinical Phenotype Prediction in Precision Medicine: A Targeted Metabolomic Analysis of Urinary Organic Acids in Autoimmune Diseases
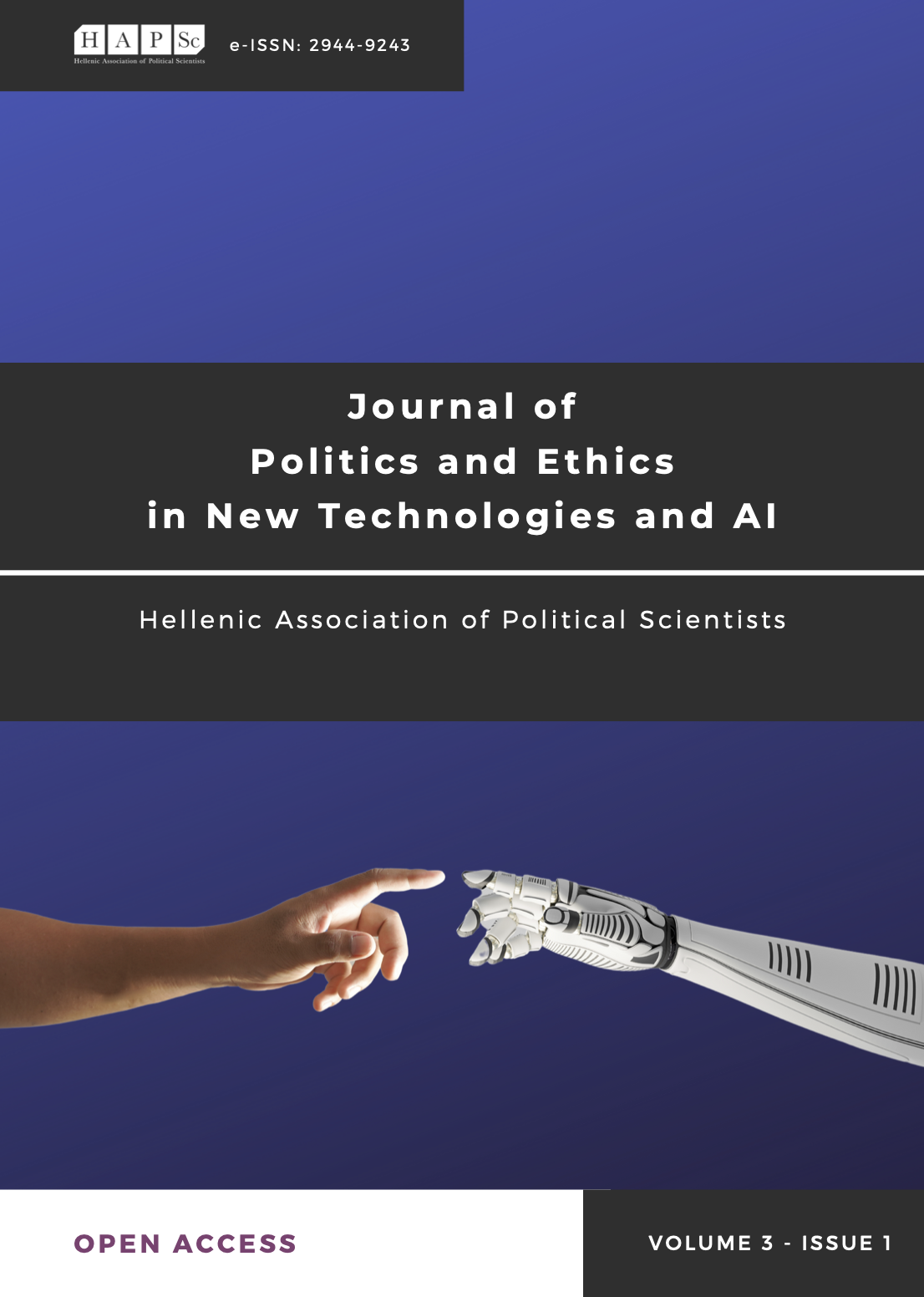
Abstract
This study aimed to create and contrast the precision of synthetic data with original data as inputs in a binary predictive feed-forward back-propagation Artificial Neural Network (ANN) for targeted analysis of urinary Organic Acids (OAs). The original dataset utilized in this analysis originated from case-control research involving 392 participants (comprising patients with autoimmune diseases and healthy individuals). Two types of synthetic data were generated using a non-parametric bootstrap replication technique and a Classification and Regression Tree (CART) model in place of the original values. Support Vector Machine (SVM) analysis was employed to pinpoint potentially crucial biomarkers for inclusion in the ANN. The accuracy of the ANN models was evaluated through the Receiver Operating Characteristic (ROC) curve, along with standard performance measurements like Sensitivity, Specificity, Positive Predicted Value, Negative Predictive Value, False Positive Rate, False Negative Rate and Overall performance. To assess the model's cross-validation and guard against overfitting, the data was randomly divided into three distinct sets: training data (50%), testing data (25%), and Holdout data (25%). The optimal architecture for all ANN models consisted of a shallow structure with one hidden layer, a hyperbolic activation function, and SoftMax as the output function. SVM analysis did not detect variations among biomarkers, indicating their equal importance. The predictive accuracy of the artificial neural network using real data was approximately 77.3%, compared to 66.6% for bootstrap-synthetic data and 51.27% for the ANN-CART model. None of the models exhibited signs of overfitting. The relatively poor performance of the ANN-CART model could be improved by adopting simpler modeling approaches and integrating alternative strategies for biomarker selection. Synthetic data quality can be enhanced through advanced statistical methodologies and may serve as a reasonable alternative for input in an ANN model while maintaining comparable accuracy in autoimmune disease prediction.
Article Details
- Section
- Research Articles

This work is licensed under a Creative Commons Attribution 4.0 International License.
Authors retain copyright and grant the journal right of first publication with the work simultaneously licensed under Creative Commons 4.0 (CC-BY 4.0) license, that allows others to share the work with an acknowledgement of the work's authorship and initial publication in this journal.