Leveraging Artificial Intelligence in the Field of Social Policy against Social Inequalities: The Current Landscape
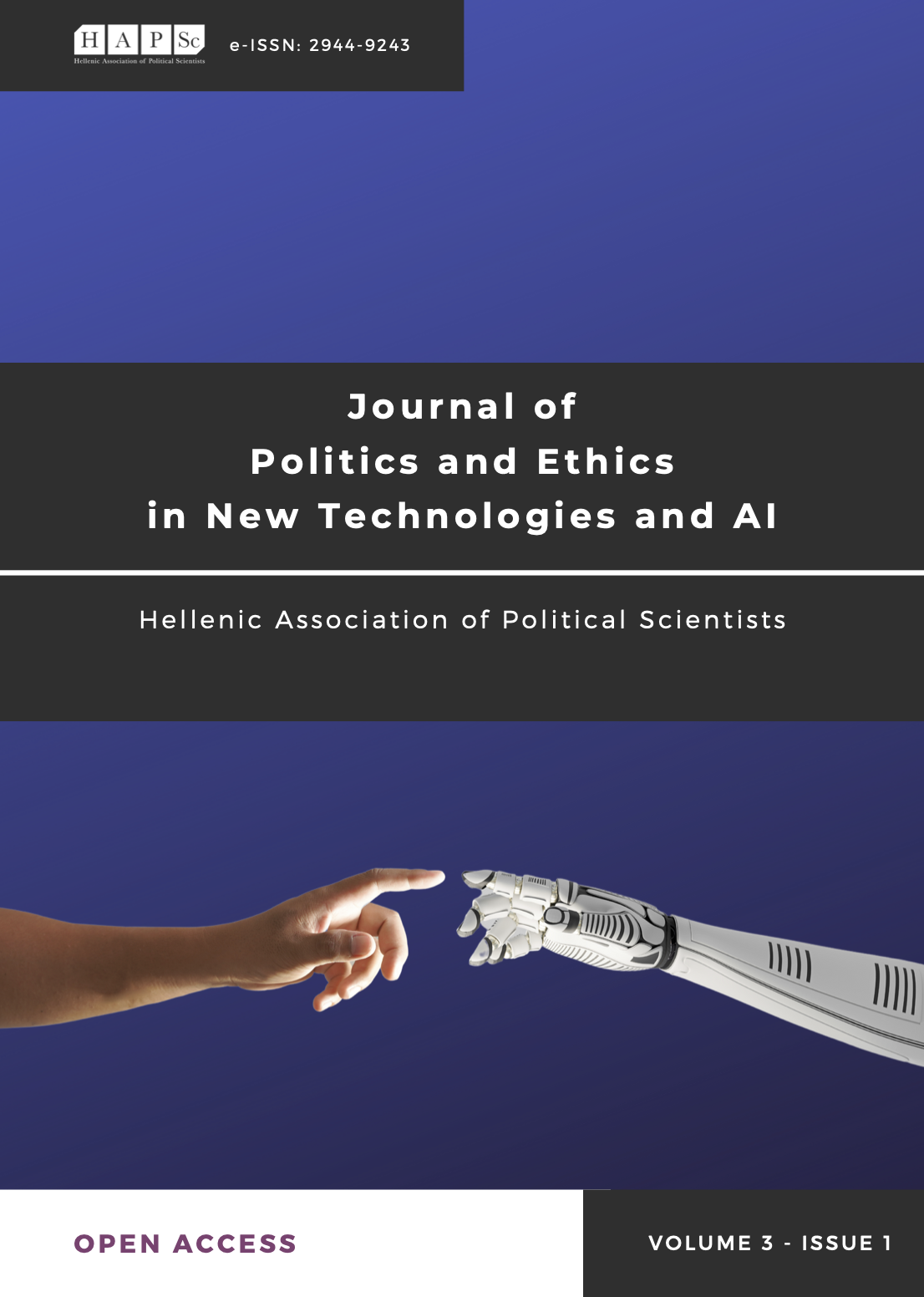
Abstract
Artificial Intelligence (AI) has become an integral part of daily human activity in both societal and political terms. Thus, it has also rapidly grown into a tool of ever-growing importance for addressing social inequalities. The increasing adoption of AI in various economical and societal aspects of daily life has significant implications for social welfare and policy development. This commentary attempts to bring the issue of the deployment of AI in social policy into the fore, examining its potential in countering and mitigating inequalities and disparities in various areas of life such as healthcare, education, and economic empowerment. There seems to be a scientific consensus that AI can be effectively utilized to mitigate social inequalities.
Article Details
- Section
- Commentaries

This work is licensed under a Creative Commons Attribution 4.0 International License.
Authors retain copyright and grant the journal right of first publication with the work simultaneously licensed under Creative Commons 4.0 (CC-BY 4.0) license, that allows others to share the work with an acknowledgement of the work's authorship and initial publication in this journal.