COMBINING FUZZY LOGIC AND INFORMATION THEORY FOR PRODUCING A LANDSLIDE SUSCEPTIBILITY MODEL
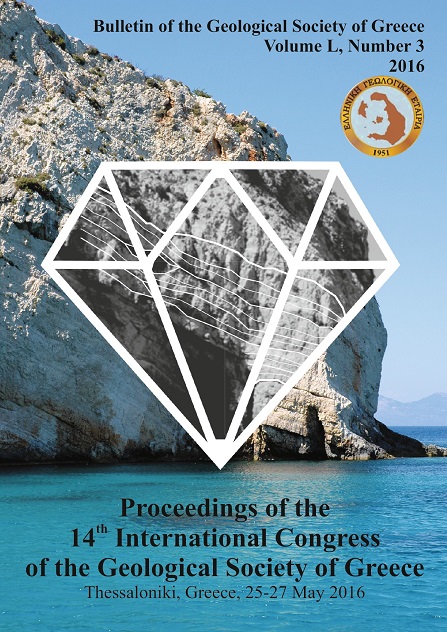
Abstract
The main objective of the present study was to develop a landslide susceptibility model by combining Fuzzy logic and Information Theory in order to estimate the spatial probability of landslide manifestation, in the mountains of central Tzoumerka, Greece. Specifically, Fuzzy logic was enabled for weighting the landslide related variables based on expert knowledge and in respect to landslide susceptibility, while the Shannon’s entropy index, an index from Information Theory, was calculated to weight the significance of each landslide related variable based on the available data. The final landslide susceptibility map was produced by applying the weighted sum method. Engineering lithological units, slope angle, slope aspect, distance from tectonic features, distance from river network and distance from road network were among the six landslide related variables that were included in the landslide database used in the training phase. The landslide inventory map was constructed by interpreting aerial photographs, satellite images and field surveys and was separated into two datasets, one for training and one for validating the model. The outcomes of the validation process illustrated that the developed methodology efficiently provided the most susceptible areas and was in good agreement with the actual landslide locations. The area under the curve was estimated to be for the training and validating datasets 0.7575 and 0.7828 respectively. The produced landslide susceptibility map could be regarded from local and national authorities as a valuable mean to evaluate strategies or to prevent and mitigate the impact of landslides. Keywords: slope stability, fuzzy weighting, Shannon’s entropy index, Tzoumerka, Greece.
Article Details
- How to Cite
-
Tsangaratos, P., & Ilia, I. (2016). COMBINING FUZZY LOGIC AND INFORMATION THEORY FOR PRODUCING A LANDSLIDE SUSCEPTIBILITY MODEL. Bulletin of the Geological Society of Greece, 50(3), 1737–1746. https://doi.org/10.12681/bgsg.11897
- Section
- Remote Sensing and GIS

This work is licensed under a Creative Commons Attribution-NonCommercial 4.0 International License.
Authors who publish with this journal agree to the following terms:
Authors retain copyright and grant the journal right of first publication with the work simultaneously licensed under a Creative Commons Attribution Non-Commercial License that allows others to share the work with an acknowledgement of the work's authorship and initial publication in this journal.
Authors are able to enter into separate, additional contractual arrangements for the non-exclusive distribution of the journal's published version of the work (e.g. post it to an institutional repository or publish it in a book), with an acknowledgement of its initial publication in this journal. Authors are permitted and encouraged to post their work online (preferably in institutional repositories or on their website) prior to and during the submission process, as it can lead to productive exchanges, as well as earlier and greater citation of published work.