A 30 year topic analysis of Veterinary Medicine literature
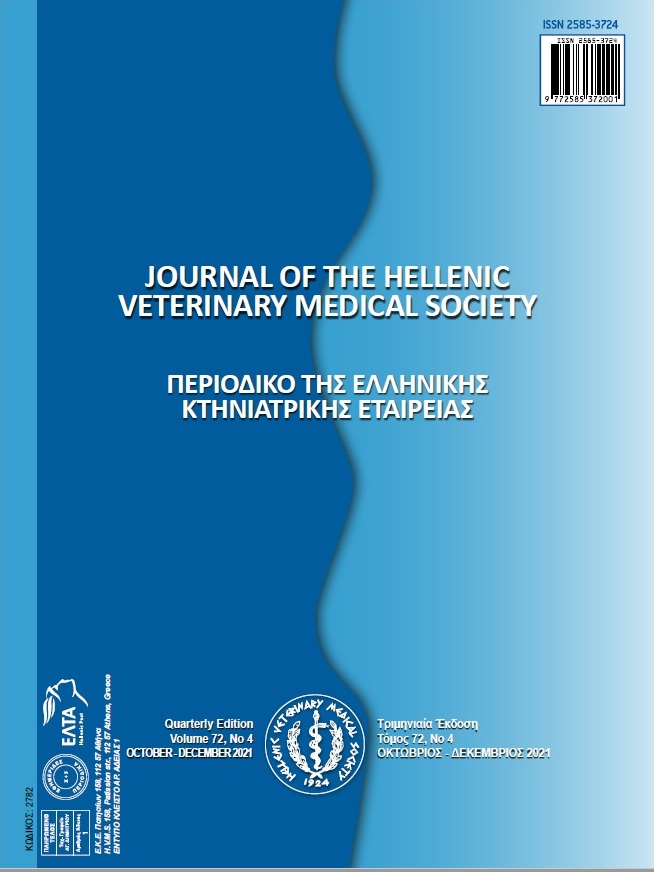
Abstract
In the present study Latent Dirichlet allocation (LDA) was used as a generative probabilistic model to extract major topics in interdecadalresearch for the Veterinary Medicine scientific literature. A total of 22 topics were extracted during the 1991-2000 period, 23 topics during 2001-2010 and 60 topics during 2011-2020. Three different algorithms were used to validate the model: perplexity, silhouette clustering and gradient boosted trees. All three validation metrics showed that LDA performed well in extracting topics. Each decade was characterized by unique topics as well as common topics which existed throughout periods. The most frequent topics were identified and trends were quantified with the use of indexes. A list of the 30 most frequent and most associated with the term Veterinary Medicine words is provided. A shift in scientific thinking probably occurred during the 30-year-period in the process of incorporating the fields related to Veterinary students, antimicrobial resistance and animals’ behavior.
Article Details
- How to Cite
-
FYTILAKOS, I., & ALEXOPOULOS, V. (2022). A 30 year topic analysis of Veterinary Medicine literature. Journal of the Hellenic Veterinary Medical Society, 72(4), 3401–3414. https://doi.org/10.12681/jhvms.29430
- Issue
- Vol. 72 No. 4 (2021)
- Section
- Research Articles
Authors who publish with this journal agree to the following terms:
· Authors retain copyright and grant the journal right of first publication with the work simultaneously licensed under a Creative Commons Attribution Non-Commercial License that allows others to share the work with an acknowledgement of the work's authorship and initial publication in this journal.
· Authors are able to enter into separate, additional contractual arrangements for the non-exclusive distribution of the journal's published version of the work (e.g. post it to an institutional repository or publish it in a book), with an acknowledgement of its initial publication in this journal.
· Authors are permitted and encouraged to post their work online (preferably in institutional repositories or on their website) prior to and during the submission process, as it can lead to productive exchanges, as well as earlier and greater citation of published work.