Seismic risk assessment of buildings and infrastructures using Artificial Neural Networks: Empirical prediction equations
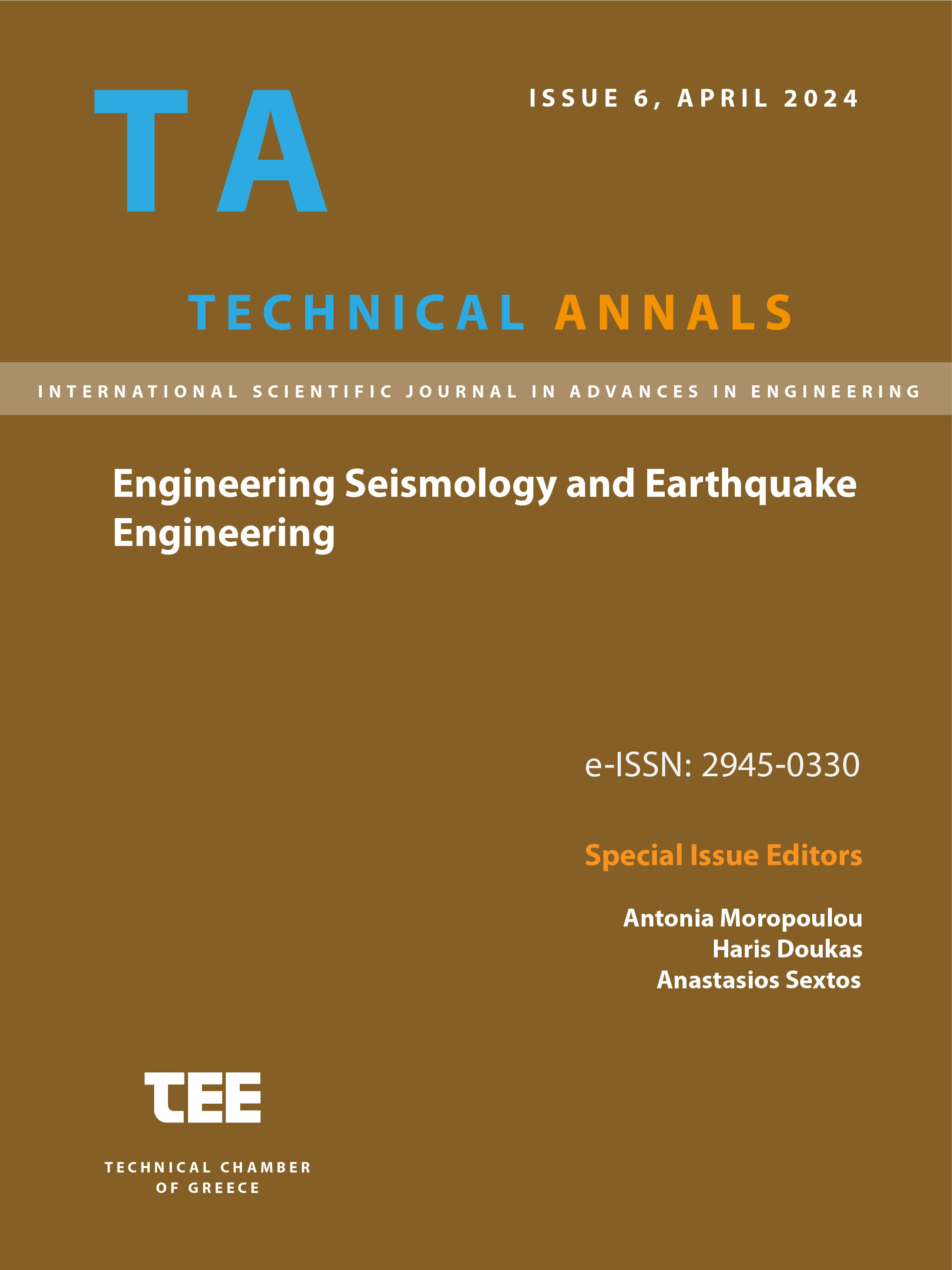
Abstract
The reliable assessment of the seismic risk, at urban, regional and national level, is extremely important for the government and society and contributes to the proper management of the pre-seismic crisis (interventions, strengthening of buildings and infrastructures), during the earthquake and post-earthquake. The seismic risk assessment involves many difficulties and uncertainties, as it depends on the successful implementation of several individual steps, starting with the identification of the elements at risk, continuing with the assessment of seismic risk and vulnerability and finishing with the estimation of the risk and losses of all types. In all the above methodological frameworks, the use of Artificial Neural Networks (ANNs) is proposed in the literature. Artificial Intelligence (AI)-based methodologies aim to improve the computational efficiency of simulations, by increasing the accuracy and reducing the computational cost. In this paper, a methodology using ANNs at the seismic hazard level is suggested to propose strong motion prediction equations (GMPE) derived from ANN training, by developing a methodological framework and a computational tool that enables continuous training and learning depending on the strong motion data that are fed to it. The proposed equations are compared with models in the literature to verify the reliability of their applicability.
Article Details
- How to Cite
-
Morfidis, K., Sotiriadis, D., Stefanidou, S., Markogiannaki, O., Karatzetzou, A., & Margaris, B. (2024). Seismic risk assessment of buildings and infrastructures using Artificial Neural Networks: Empirical prediction equations. Technical Annals, 1(6). https://doi.org/10.12681/ta.36895
- Section
- Artificial Intelligence

This work is licensed under a Creative Commons Attribution-NonCommercial-ShareAlike 4.0 International License.