The application of data science and machine learning techniques in predicting the compressive strength of confined concrete
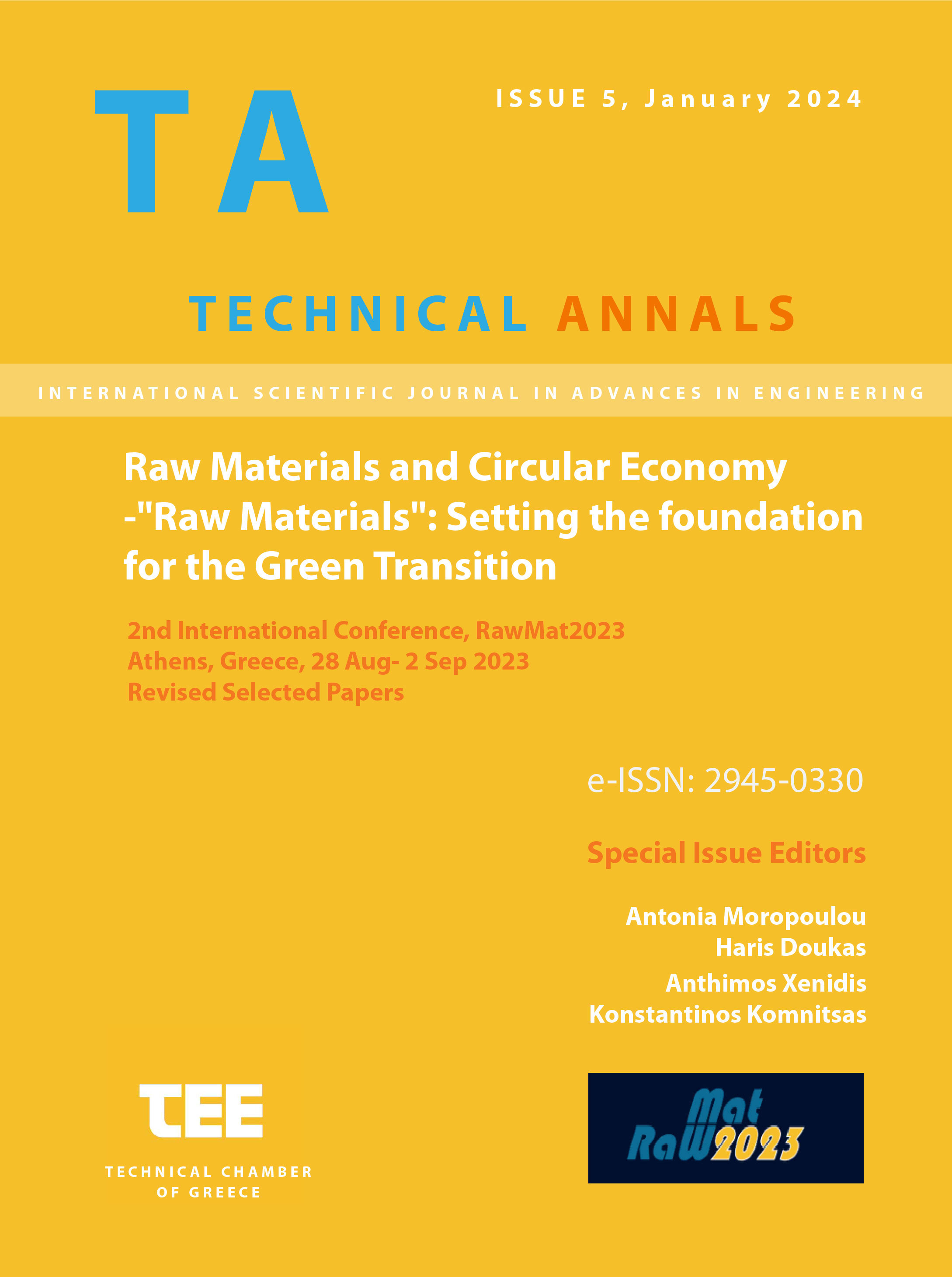
Abstract
The integration of machine learning (ML) techniques into industrial and manufacturing applications has seen great growth in recent years. Various numerical and analytical models have been proposed, based either on experimental results or simulation results, and have helped to understand phenomena that take place during the life cycle of a material. In this direction, a large experimental data set to determine the compressive strength of FRP (FRP) prestressed concrete specimens has been used as a basis in this work.
The obtained measurements are correlated with the mechanical and structural properties of the material and fed into a ML model. The model is trained on the experimental values and can provide predictions for conditions within or outside the value range of the input data. Various MLalgorithms are implemented and studied for their prediction accuracy, and the results show that ML can be an important computational tool, which can act as a complement to expensive experiments or time-consuming simulations in engineering sciences.
Article Details
- How to Cite
-
Papakonstantinou, C., Valasaki, M., Sofos, F., & Karakasidis, T. (2024). The application of data science and machine learning techniques in predicting the compressive strength of confined concrete. Technical Annals, 1(5). https://doi.org/10.12681/ta.37190
- Section
- Material Science and Engineering

This work is licensed under a Creative Commons Attribution-NonCommercial-ShareAlike 4.0 International License.