A study of the performance of the MSR vegetation index, using probabilistic and geostatistical methods
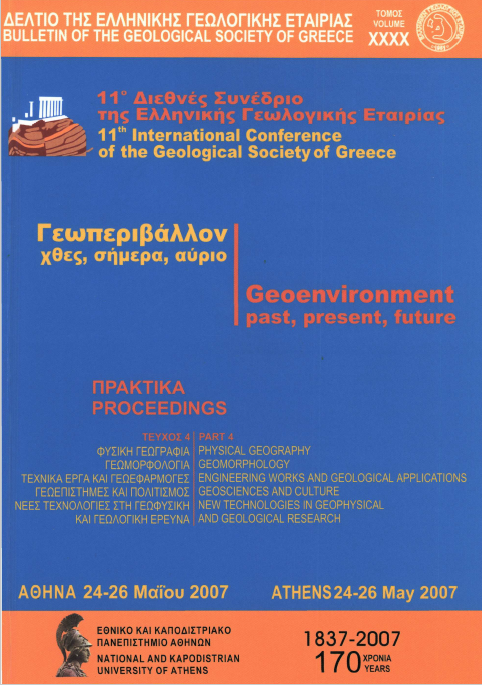
Abstract
In the present paper is studied the effect of the MSR (Modified Soil Ratio) vegetation index on multispectral digital images, with the aid of probability theory and geostatistics. Using proper distributions to describe the histograms of the image at the red and infrared band zones, an analytical expression of the distribution g of the MSR values is deduced. The study of the behaviour of g shows that the ratio of the standard deviation to the mean value of the MSR image is higher than that of the NDVI vegetation index, which is quite often used. This means that the MSR vegetation index produces images with a good contrast. It was also observed that the MSR image has a better signal to noise ratio than that of the NDVI image. Finally, the autocorrelograms of the MSR and NDVI images showed that the tonality differences between adjacent pixels of the MSR image are slightly stronger than those of the NDVI image. The general conclusion is that the MSR vegetation index produces images with a good contrast and a high signal to noise ratio, which could aid in making a reliable mapping of the vegetation cover of the area under study
Article Details
- How to Cite
-
Skianis, A. G., Vaiopoulos, D., & Nikolakopoulos, K. (2007). A study of the performance of the MSR vegetation index, using probabilistic and geostatistical methods. Bulletin of the Geological Society of Greece, 40(4), 2048–2059. https://doi.org/10.12681/bgsg.17297
- Section
- New Technologies in Geophysical and Geological Research

This work is licensed under a Creative Commons Attribution-NonCommercial 4.0 International License.
Authors who publish with this journal agree to the following terms:
Authors retain copyright and grant the journal right of first publication with the work simultaneously licensed under a Creative Commons Attribution Non-Commercial License that allows others to share the work with an acknowledgement of the work's authorship and initial publication in this journal.
Authors are able to enter into separate, additional contractual arrangements for the non-exclusive distribution of the journal's published version of the work (e.g. post it to an institutional repository or publish it in a book), with an acknowledgement of its initial publication in this journal. Authors are permitted and encouraged to post their work online (preferably in institutional repositories or on their website) prior to and during the submission process, as it can lead to productive exchanges, as well as earlier and greater citation of published work.