Using AI Changes the Paradigm of Women's Participation in Politics
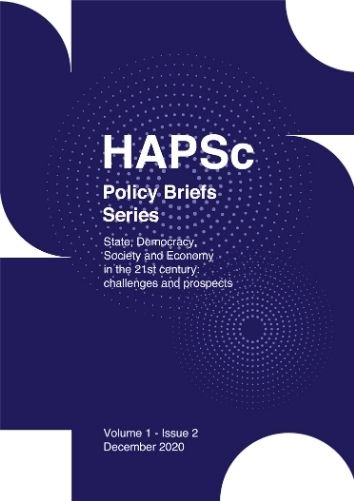
Abstract
The effect of AI on how people are viewed and handled in society is important and profound. However, a vicious cycle is maintained with AI's algorithms design and implementation. Among others, predictive models, machine learning and AI algorithms train and test themselves using datasets, as a result, they “learn” mainly based on the data input in a model. Nowadays and in this context, it seems that there is a growing scientific dialogue concerning bias in training AI (Falco, 2019; Lu, 2019; Straw, 2020) as well as whether datasets, on which decisions are made, only represent fractions of reality (Günther et al., 2017). The technology often captures and reproduces regulated and restrictive beliefs regarding gender and race, which are then repetitively strengthened: Gender relations be materialized by inventions and, through their enrolment and incorporation of machinery, masculinity, and femininity gain of turn their importance and character. When robots progress in certain cognitive functions, their comparatively weak abilities will definitely get better. This list incorporates the innovative approach to the dilemma, empathy, negotiation, and belief. Automation and AI will also replace many of today's workers at the same time creating new opportunities for specialized personnel– so that is why women need to get into this emerging sector and ensure that they can secure new jobs when their jobs are squeezed. In addition, AI may provide the ability to alter male and female epistemological assumptions. The narration of "hard" and "soft" intelligence, for instance, is often described as male and female. The rise and development of AI is also seen as pushing economic growth and strengthening political influence. In politics, UK politics still dominates the ambition of economic development by technical advancement. Jude Browne states (Clementine Collett & Sarah Dillon) that a national AI agency equivalent to Human Fertilization and Embryology (HFEA) has yet to be set up by the government of the UK that will fill the divide between national, experts and government, for example. Browne claims that it includes the dominance, primarily guided by the goals of economic wealth, of private interest over the public interest. There is a possibility that economic growth and political influence play an important part in influencing AI laws and policies at the cost of other motivations, which are more morally equal. Consequently, a dual-purpose must be incorporated into an equitable AI policy. Firstly, to ensure there is no rise in social and economic disparity due to the advancement of AI technology. Secondly, to call AI to cut this down. AI must first and foremost enable us to promote our democratic liberties, enhance social harmony, and enhance unity, rather than jeopardise our individual trajectories and networks of solidarity.
Article Details
- How to Cite
-
Efthymiou, I. .-. P., Psomiadi, A., Diareme, K. C., & Chatzivasileiou, S. (2020). Using AI Changes the Paradigm of Women’s Participation in Politics. HAPSc Policy Briefs Series, 1(2), 26–34. https://doi.org/10.12681/hapscpbs.26479
- Section
- Articles

This work is licensed under a Creative Commons Attribution 4.0 International License.
Authors retain copyright and grant the journal right of first publication with the work simultaneously licensed under a Creative Commons Attribution License that allows others to share the work with an acknowledgement of the work's authorship and initial publication in this journal.