AI and Big Data: A New Paradigm for Decision Making in Healthcare
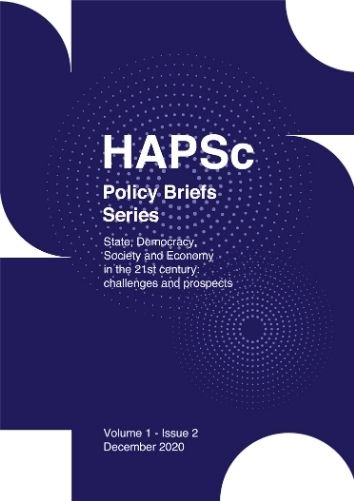
Abstract
The latest developments in artificial intelligence (AI)—a general-purpose technology impacting many industries — have been based on advancements in machine learning, which is recast as a quality-adjusted decline in forecasting ratio. The influence of Policy on AI and big data has impacted two key magnitudes which are known as diffusion and consequences. And these must be focused primarily on the context of AI and big data. First, in addition to the policies on subsidies and intellectual property (IP) that will affect the propagation of AI in ways close to their effect on other technologies, three policy categories — privacy, exchange, and liability — may have a specific impact on the diffusion of AI. The first step in the prohibition process is to identify the shortcomings of current hospital procedures, why we need acute care AI, and eventually how the direction of patient decision-making will shift with the introduction of AI-based research. The second step is to establish a plan to shift the direction of medical education in order to enable physicians to retain control of AI. Medical research would need to rely less on threshold decision-making and more on the prediction, interpretation, and pathophysiological context of contextual time cycles. This should be an early part of a medical student's education, and this is what their hospital aid (AI) ought to do. Effective contact between human and artificial intelligence includes a shared pattern of focused knowledge base. Human-to-human contact protection in hospitals should lead this professional transformation process.
Article Details
- How to Cite
-
Efthymiou, I. .-. P., Vozikis, A., Sidiropoulos, S., & Kritas, D. (2020). AI and Big Data: A New Paradigm for Decision Making in Healthcare. HAPSc Policy Briefs Series, 1(2), 138–145. https://doi.org/10.12681/hapscpbs.26490
- Section
- Articles

This work is licensed under a Creative Commons Attribution 4.0 International License.
Authors retain copyright and grant the journal right of first publication with the work simultaneously licensed under a Creative Commons Attribution License that allows others to share the work with an acknowledgement of the work's authorship and initial publication in this journal.