Annealing temperatures affect 16S rRNA gene-amplicon Illumina sequencingbased bacterial community analysis of canine skin
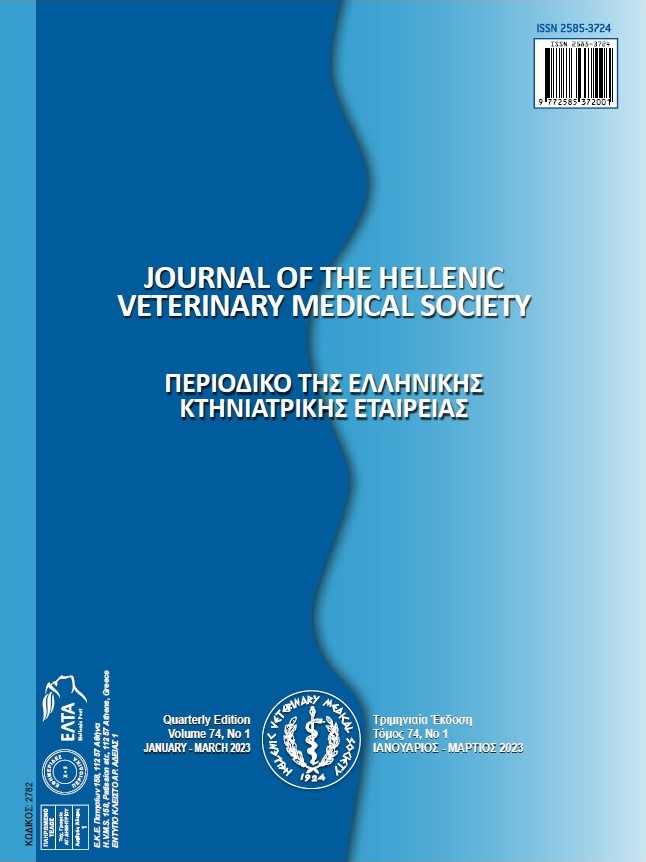
Abstract
Analysis of the bacterial community structure of dog skin samples, sequencing the 16S rRNA gene is nowadays widely used. Among others, the 16S rRNA gene amplicon Illumina sequencing technique is well established and routinely applied to get a first inside into the bacterial community diversity and taxonomic composition. However, as it is a molecular-based technique, bias due to methodology is possible and should be minimized. In this study, we tested the effects of annealing temperature (50°C vs 55°C) on the 16S rRNA gene amplicon analysis of the bacterial microbiota of skin and ear canal samples from a German shepherd dog. Although beta diversity was not affected by the higher annealing temperature, alpha diversity values showed a shift (overall diversity (Shannon) and evenness were increased, whereas dominance (D), number of taxa (S), richness (Chao 1) and the total numbers of individuals (N) were reduced, with higher annealing temperature). The biological relevance of this finding remains unclear. Thus, our results underline the importance of optimal annealing temperature in order to minimize bias, as well as the necessity of further similar studies with a larger sample size.
Article Details
- Zitationsvorschlag
-
Apostolopoulos, N., Thom, N., Bagwe, R., Müller, R., Ewers, C., & Glaeser, P. (2023). Annealing temperatures affect 16S rRNA gene-amplicon Illumina sequencingbased bacterial community analysis of canine skin. Journal of the Hellenic Veterinary Medical Society, 74(1), 5371–5378. https://doi.org/10.12681/jhvms.29577
- Ausgabe
- Bd. 74 Nr. 1 (2023)
- Rubrik
- Short Communication

Dieses Werk steht unter der Lizenz Creative Commons Namensnennung - Nicht-kommerziell 4.0 International.
Authors who publish with this journal agree to the following terms:
· Authors retain copyright and grant the journal right of first publication with the work simultaneously licensed under a Creative Commons Attribution Non-Commercial License that allows others to share the work with an acknowledgement of the work's authorship and initial publication in this journal.
· Authors are able to enter into separate, additional contractual arrangements for the non-exclusive distribution of the journal's published version of the work (e.g. post it to an institutional repository or publish it in a book), with an acknowledgement of its initial publication in this journal.
· Authors are permitted and encouraged to post their work online (preferably in institutional repositories or on their website) prior to and during the submission process, as it can lead to productive exchanges, as well as earlier and greater citation of published work.