Fishing the waves: comparing GAMs and random forest to evaluate the effect of changing marine conditions on the energy performance of vessels
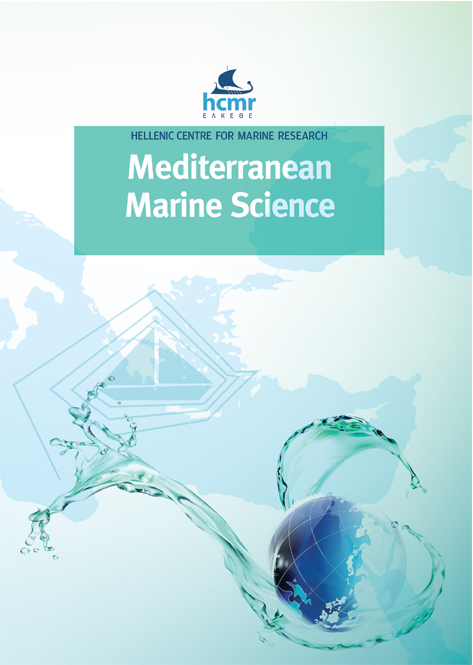
Abstract
The optimization of consumption and the reduction of gas emissions in fisheries rely on a thorough examination of all factors affecting the energy balance of fishing vessels. Engines, propellers, or the hydrodynamic characteristics of vessels and gears are unquestionably the primary factors affecting this balance, and an improvement in energy efficiency based on these factors is typically attained through technical modifications to existing technologies. Behavioral modifications, such as a reduction in operational speeds or the selection of closer fishing grounds, are another option. There may still be room for improvement in behavioral responses, for instance by adapting fishing strategies in response to changing weather and sea conditions. As far as the authors are aware, the influence of varying sea state and wind conditions on the energy expenditure of fishing vessels has not yet been investigated and is the focus of this research. In this study, wind and wave actions were associated with the observed activity of three fishing vessels operating in the northern Adriatic Sea: an OTB, a PTM, and a TBB trawler. The analysis made use of a comparison between two different approaches, generalized additive models (GAMs) and random forest, in order to quantify the significance of each variable on the response and generate consumption forecasts. In our analysis, the observed influence of predictors was significant albeit occasionally ambiguous. Wave height had the most obvious impact on energy expenditure, with the towing and gear handling phases being the most affected by wave action. Conversely, navigation seemed to be mostly unaffected by significant wave heights up to 1.5 meters, with unclear effects on consumption estimated above this threshold. The relationship between winds and fuel consumption was found to be nonlinear and ambiguous; hence, its significance should be investigated further.
Article Details
- How to Cite
-
COLOMBELLI, A., PULCINELLA, J., BONANOMI, S., NOTTI, E., MORO, F., & SALA, A. (2022). Fishing the waves: comparing GAMs and random forest to evaluate the effect of changing marine conditions on the energy performance of vessels. Mediterranean Marine Science, 23(4), 935–951. https://doi.org/10.12681/mms.29721
- Section
- Research Article
Authors who publish with this journal agree to the following terms:
- Authors retain copyright and grant the journal right of first publication with the work simultaneously licensed under a Creative Commons Attribution Non-Commercial License that allows others to share the work with an acknowledgement of the work's authorship and initial publication in this journal.
- Authors are able to enter into separate, additional contractual arrangements for the non-exclusive distribution of the journal's published version of the work (e.g. post it to an institutional repository or publish it in a book), with an acknowledgement of its initial publication in this journal.
- Authors are permitted and encouraged to post their work online (preferably in institutional repositories or on their website) prior to and during the submission process, as it can lead to productive exchanges, as well as earlier and greater citation of published work (See The Effect of Open Access).