Χρησιμοποιήστε έναν δείκτη γενετικής τροποποίησης για να αυξήσετε την ακρίβεια και την ακρίβεια των γονιδιωματικών αναλύσεων
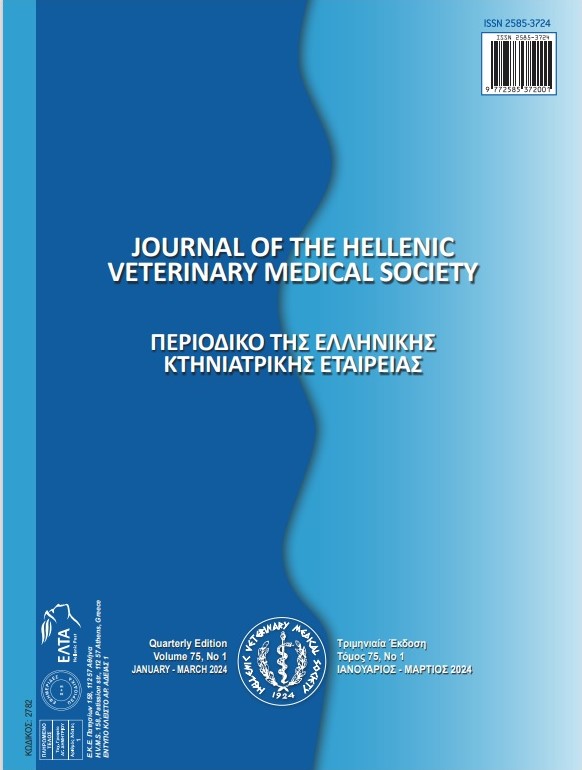
Περίληψη
Το υψηλό κόστος προσδιορισμού του γονότυπου και η χαμηλή ακρίβεια της αξιολόγησης σε μικρό αριθμό δειγμάτων γονότυπου όταν χρησιμοποιούνται γονιδιωματικά δεδομένα και γενετικοί δείκτες για την εκτέλεση γονιδιωματικών αξιολογήσεων είναι δύο σημαντικά προβλήματα. Η επίδραση των επιπέδων γενετικών δεικτών σε έναν πληθυσμό F2 που ελήφθη από αμφίδρομη διασταύρωση ιθαγενών κοτόπουλου Ιράν με χαμηλό ρυθμό ανάπτυξης και στελέχους κρέατος Arian με υψηλό ρυθμό ανάπτυξης διερευνήθηκε σε αυτή τη μελέτη προκειμένου να απομονωθούν SNPs με υψηλότερη επίδραση και να χρησιμοποιηθούν αυτοί οι δείκτες στη γονιδιωματική αξιολόγηση ως κατάλληλη μέθοδος διαλογής SNPs προκειμένου να αυξηθεί η ακρίβεια της αξιολόγησης και να μειωθεί το κόστος γονότυπου. Σε αυτή τη μελέτη, μελετήθηκε η ακρίβεια πρόβλεψης των τιμών διόρθωσης σε πέντε ομάδες δεικτών με διάφορα MAF, επιπλέον της απόδειξης της υπεροχής της προσέγγισης ssGBLUP έναντι της μεθόδου BLUP από τη μέθοδο 5-πλής διασταυρούμενης επικύρωσης (CV) στο ένα βήμα στρατηγική αξιολόγησης. Αυτή η ομάδα δεικτών (MAF 0,4 - 0,5) εισήχθη ως το καλύτερο επίπεδο αλληλικής συχνότητας για τη διεξαγωγή γονιδιωματικών αξιολογήσεων για το χαρακτηριστικό ανάπτυξης αφού τα αποτελέσματα έδειξαν ότι η χρήση SNPs με αλληλική συχνότητα 0,4-0,5 σε καθεμία από τη δεύτερη έως την έβδομη εβδομάδα έδειξε υψηλότερη προγνωστική ακρίβεια από τις πληροφορίες όλων των SNP. Εκτός από την επιβολή χαμηλού κόστους γονότυπου, η χρήση SNP με αλληλική συχνότητα 0,4–0,5 και η ανάπτυξη τσιπ SNP χαμηλής πυκνότητας με δείκτες με τις προαναφερθείσες ιδιότητες μπορεί να χρησιμοποιηθεί για την αξιόπιστη αξιολόγηση ατόμων με βάση τη γενετική αξία.
Λεπτομέρειες άρθρου
- Πώς να δημιουργήσετε Αναφορές
-
Safarzdeh Μ., Kalani, M., Νιζαφάτ α., Χωρίς M., Ghadermarzi Ν. γ. κ., το ετοιμάζω Ο. Χ., Ρατζάμπι Ο. Σ., & Pish Jang Aghajeri Τ. (2024). Χρησιμοποιήστε έναν δείκτη γενετικής τροποποίησης για να αυξήσετε την ακρίβεια και την ακρίβεια των γονιδιωματικών αναλύσεων. Περιοδικό της Ελληνικής Κτηνιατρικής Εταιρείας, 75(2), 7537–7550. https://doi.org/10.12681/jhvms.35220 (Original work published 16 Ιούλιος 2024)
- Τεύχος
- Τόμ. 75 Αρ. 2 (2024)
- Ενότητα
- Research Articles

Αυτή η εργασία είναι αδειοδοτημένη υπό το CC Αναφορά Δημιουργού – Μη Εμπορική Χρήση 4.0.
Οι συγγραφείς των άρθρων που δημοσιεύονται στο περιοδικό διατηρούν τα δικαιώματα πνευματικής ιδιοκτησίας επί των άρθρων τους, δίνοντας στο περιοδικό το δικαίωμα της πρώτης δημοσίευσης.
Άρθρα που δημοσιεύονται στο περιοδικό διατίθενται με άδεια Creative Commons 4.0 Non Commercial και σύμφωνα με την άδεια μπορούν να χρησιμοποιούνται ελεύθερα, με αναφορά στο/στη συγγραφέα και στην πρώτη δημοσίευση για μη κερδοσκοπικούς σκοπούς.
Οι συγγραφείς μπορούν να καταθέσουν το άρθρο σε ιδρυματικό ή άλλο αποθετήριο ή/και να το δημοσιεύσουν σε άλλη έκδοση, με υποχρεωτική την αναφορά πρώτης δημοσίευσης στο J Hellenic Vet Med Soc
Οι συγγραφείς ενθαρρύνονται να καταθέσουν σε αποθετήριο ή να δημοσιεύσουν την εργασία τους στο διαδίκτυο πριν ή κατά τη διαδικασία υποβολής και αξιολόγησής της.