Computational Model of Intelligence
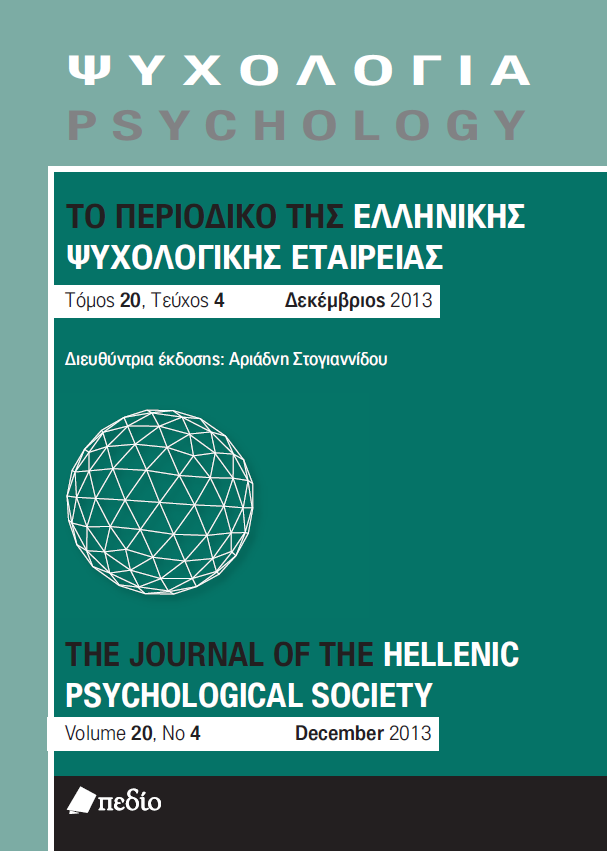
Abstract
The purpose of this very study is to highlight important issues underlying the concept of intelligence. Human intelligence has been a crucial topic of heated debate over the years and, as a result, the very nature and structure of intelligence still remain vague. This research addresses intelligence in terms of three aspects namely processing speed, speed of learning and the representational power required to solve complex intellectual tasks. Although all these have been suggested as candidate mechanisms of intelligence, the correlation between them as well as their impact on cognition is still unclear. To investigate this even further, an artificial neural network was developed in Matlab, in order to provide a mechanistic approach to intelligence. This artificial neural network was used to test out the hypothesis that certain parameter manipulations, such as altering the activation function, learning rate or the number of hidden units in the network, could be linked to the network’s processing speed, speed of learning and representational power. Results suggest that only modifications in
the learning rate produced such changes across the aforementioned three constructs. As a result, this parameter is suggested as a candidate mechanism that underlies an important portion of the neurocomputational basis of human intelligence. The implications are discussed.
Article Details
- How to Cite
-
Πανούτσος Σ. .-. Ε. (2020). Computational Model of Intelligence. Psychology: The Journal of the Hellenic Psychological Society, 20(4), 448–461. https://doi.org/10.12681/psy_hps.23602
- Issue
- Vol. 20 No. 4 (2013)
- Section
- RESEARCH PAPERS

This work is licensed under a Creative Commons Attribution-ShareAlike 4.0 International License.
The journal PSYCHOLOGY adopts a Platinum open-access policy. Submission, processing or publication costs are waived by the Hellenic Psychological Society. Papers published in the journal PSYCHOLOGY are licensed under a 'Creative Commons Attribution-ShareAlike 4.0 International' licence. The authors reserve the copyright of their work and grant the journal the right of its first publication. Third-party licensees are allowed to use the published paper immediately after publication as they wish, provided they retain the defined by the license copyright formalities, regarding the reference to its author(s) and its initial publication in the journal PSYCHOLOGY. Moreover, any adjusted work should be shared under the same reuse rights, so with the same CC license.