The role of Spatial Autocorrelation on spatially correlated data for Hierarchical Cluster Analysis
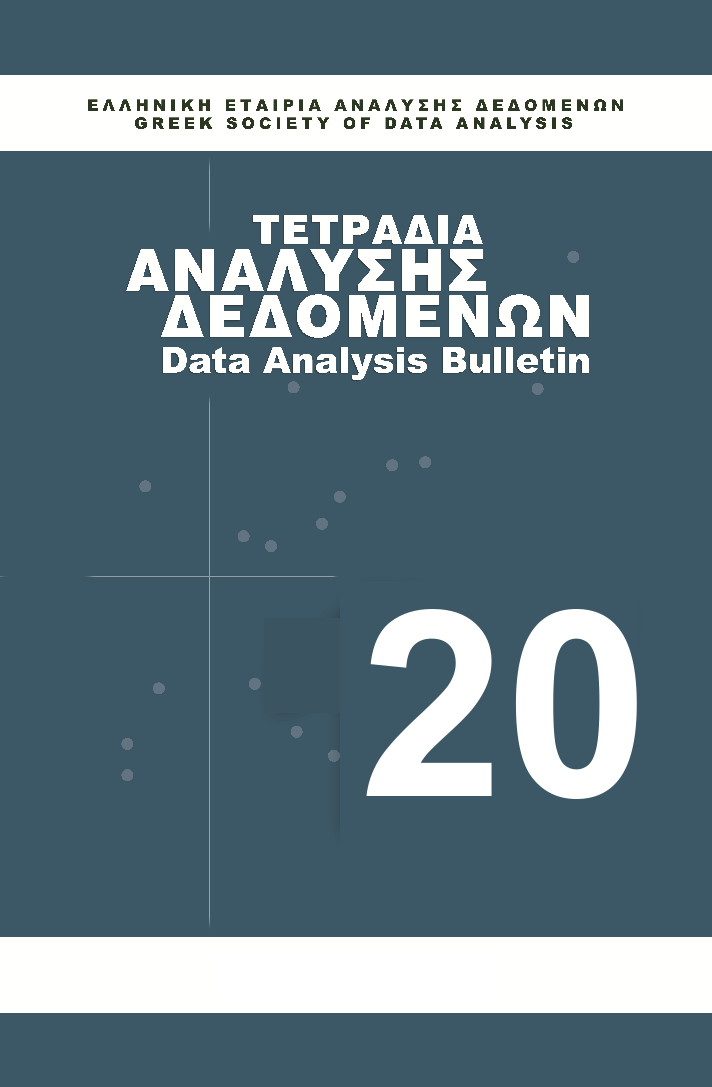
Περίληψη
Λεπτομέρειες άρθρου
- Πώς να δημιουργήσετε Αναφορές
-
Koutsos, T., & Menexes, G. (2024). The role of Spatial Autocorrelation on spatially correlated data for Hierarchical Cluster Analysis. Τετράδια Ανάλυσης Δεδομένων, 20(1), 109–119. ανακτήθηκε από https://ejournals.epublishing.ekt.gr/index.php/dab/article/view/33519
- Ενότητα
- Μεθοδολογικές προσεγγίσεις

Αυτή η εργασία είναι αδειοδοτημένη υπό το CC Αναφορά Δημιουργού – Μη Εμπορική Χρήση – Όχι Παράγωγα Έργα 4.0 4.0.
Οι Συγγραφείς που δημοσιεύουν εργασίες τους στο περιοδικό ΤΕΤΡΑΔΙΑ ΑΝΑΛΥΣΗΣ ΔΕΔΟΜΕΝΩΝ συμφωνούν στους παρακάτω όρους:
- Οι Συγγραφείς δεν χρεώνονται με έξοδα υποβολής, επεξεργασίας ή δημοσίευσης των εργασιών τους. Τα κόστη αυτά καλύπτονται από την Ελληνική Εταιρία Ανάλυσης Δεδομένων.
- Τα πνευματικά δικαιώματα των εργασιών που δημοσιεύονται στο περιοδικό ΤΕΤΡΑΔΙΑ ΑΝΑΛΥΣΗΣ ΔΕΔΟΜΕΝΩΝ προστατεύονται από την άδεια Creative Commons Attribution-NonCommercial-ΝοDerivatives 4.0 International. Οι Συγγραφείς διατηρούν τα Πνευματικά Δικαιώματα και χορηγούν στο περιοδικό το δικαίωμα της πρώτης δημοσίευσης. Η άδεια αυτή επιτρέπει σε τρίτους - αποδέκτες της άδειας να χρησιμοποιούν την εργασία σε οποιαδήποτε μορφή μόνο για μη εμπορικούς σκοπούς. Αν οι τρίτοι τροποποιήσουν ή προσαρμόσουν το περιεχόμενο, οφείλουν να κοινοποιήσουν το τροποποιημένο περιεχόμενο for noncommercial purposes only. If others modify or adapt the material, they must license the modified material under identical terms.
- Με την προϋπόθεση της διατήρησης των διατυπώσεων που προβλέπονται στην άδεια σχετικά με την αναφορά στον αρχικό δημιουργό και την αρχική δημοσίευση στο περιοδικό ΤΕΤΡΑΔΙΑ ΑΝΑΛΥΣΗΣ ΔΕΔΟΜΕΝΩΝ.
- Οι Συγγραφείς μπορούν να συνάπτουν ξεχωριστές και πρόσθετες συμβάσεις και συμφωνίες για τη μη αποκλειστική διανομή της εργασίας όπως δημοσιεύτηκε στο περιοδικό ΤΕΤΡΑΔΙΑ ΑΝΑΛΥΣΗΣ ΔΕΔΟΜΕΝΩΝ (π.χ. κατάθεση σε ακαδημαϊκά καταθετήρια), με την προϋπόθεση της αναγνώρισης και την αναφοράς της πρώτης δημοσίευσης στο περιοδικό ΤΕΤΡΑΔΙΑ ΑΝΑΛΥΣΗΣ ΔΕΔΟΜΕΝΩΝ.
- Το περιοδικό ΤΕΤΡΑΔΙΑ ΑΝΑΛΥΣΗΣ ΔΕΔΟΜΕΝΩΝ Α επιτρέπει και ενθαρρύνει τους συγγραφείς να καταθέτουν τις εργασίες τους σε θεσμικά (π.χ. το αποθετήριο του Εθνικού Κέντρου Τεκμηρίωσης) ή θεματικά αποθετήρια, μετά τη δημοσίευσή τους στο ΤΕΤΡΑΔΙΑ ΑΝΑΛΥΣΗΣ ΔΕΔΟΜΕΝΩΝ και με όρους Ανοικτής Πρόσβασης, όπως κατά περίπτωση προσδιορίζονται από τους χρηματοδότες της έρευνάς τους ή/και τα ιδρύματα με τα οποία συνεργάζονται. Κατά την κατάθεση της εργασίας τους, οι συγγραφείς πρέπει να παρέχουν πληροφορίες σχετικά με τη δημοσίευση της εργασίας στο περιοδικό και τις πηγές χρηματοδότησης της έρευνάς τους. Κατάλογοι των ιδρυματικών και θεματικών αποθετηρίων ανά χώρα υπάρχουν στη βάση http://opendoar.org/countrylist.php. Οι συγγραφείς έχουν τη δυνατότητα να καταθέσουν χωρίς κόστος την εργασία τους στο αποθετήριο www.zenodo.org, το οποίο υποστηρίζεται από το OpenAIRE (www.openaire.eu ), στο πλαίσιο των πολιτικών της Ευρωπαϊκής Επιτροπής για την ενίσχυση της Ανοικτής ακαδημαϊκής έρευνας.