The <<interpretive plane>> in the interpretation of the factorial plane with supplementary variables: Application to Financial Data Analytics
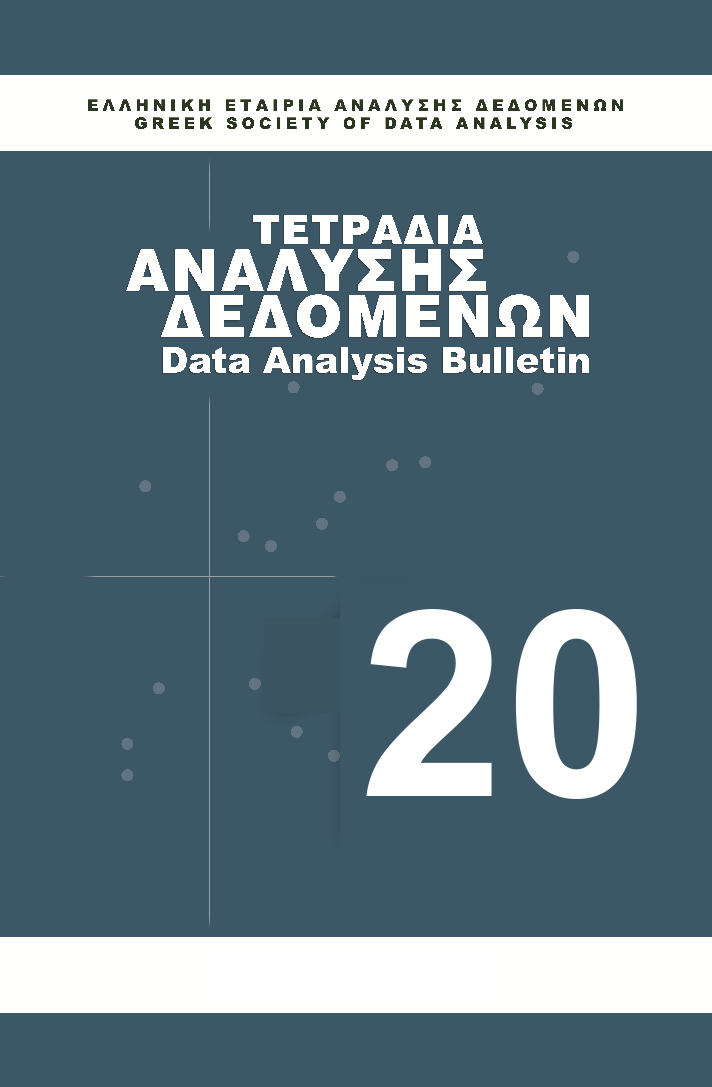
Abstract
Multiple Correspondence Analysis (MCA) is a method of unsupervised machine learning applied to categorical data in order to investigate the associations between variable categories, as well as the directions (trends) of their dispersion. The so-called supplementary variables, also used in the context of ΜCA, do not participate in the construction of the factorial axes, are simply projected through the transition formulas together with the other points (categories of variables) on the factorial diagrams, and can further contribute to the interpretation of the phenomenon under study. This paper examines the way in which graphical information can be integrated from the "Interpretive Plane" into the factorial planes that include supplementary points. Through the Interpretive Plane, the important interpretive points of the factorial plane are identified, and then those that are "close" to supplementary points. Thus, practitioners who wish to include supplementary variables in their analyses can achieve better and safer interpretative results-conclusions. The proposed approach was applied to the investigation of the rewards system, the performance, development and effectiveness of businesses. The business sector variables and the hierarchical position of employees in the enterprise were considered as supplementary.
Article Details
- How to Cite
-
Moschidis, S., & Thanopoulos, A. C. (2024). The <<interpretive plane>> in the interpretation of the factorial plane with supplementary variables: Application to Financial Data Analytics. Data Analysis Bulletin, 20(1), 24–31. Retrieved from https://ejournals.epublishing.ekt.gr/index.php/dab/article/view/32333
- Section
- Empirical studies

This work is licensed under a Creative Commons Attribution-NonCommercial-NoDerivatives 4.0 International License.
Authors who publish their work in the journal DATA ANALYSIS BULLETIN agree to the following terms:
1. Authors will not be charged any submission, processing or publication fees for their work. These costs are covered by the Greek Society of Data Analysis.
2. The copyright of papers published in the journal DATA ANALYSIS BULLETIN is protected by the Creative Commons Attribution-NonCommercial-NoDerivatives 4.0 International license. The Authors retain the Copyright and grant the journal the right of first publication. This license allows third party licensees to use the work in any form for non-commercial purposes only. If third parties modify or adapt the content, they must license the modified material for noncommercial purposes only. If others modify or adapt the material, they must license the modified material under identical terms.
3. Provided that the terms of the licence concerning the reference to the original author and the original publication in the journal DATA ANALYSIS BULLETIN are maintained.
4. Authors may enter into separate and additional contracts and agreements for the non-exclusive distribution of the work as published in the DATA ANALYSIS BULLETIN journal (e.g., deposit in academic repositories), provided that the acknowledgement and citation of the first publication in the DATA ANALYSIS BULLETIN journal is acknowledged.
5. The DATA ANALYSIS BULLETIN journal allows and encourages authors to deposit their work in institutional (e.g. the repository of the National Documentation Centre) or thematic repositories, after publication in DATA ANALYSIS BULLETIN and under Open Access conditions, as determined by their research funders and/or the institutions with which they collaborate, as appropriate. When submitting their work, authors should provide information on the publication of the work in the journal and the sources of funding for their research. Lists of institutional and thematic repositories by country are available at http://opendoar.org/countrylist.php. Authors can deposit their work free of charge in the repository www.zenodo.org, which is supported by OpenAIRE (www.openaire.eu ), as part of the European Commission's policies to support Open Academic Research.