Using Data Analytics methods before using Machine Learning algorithms: prediction on mixed data
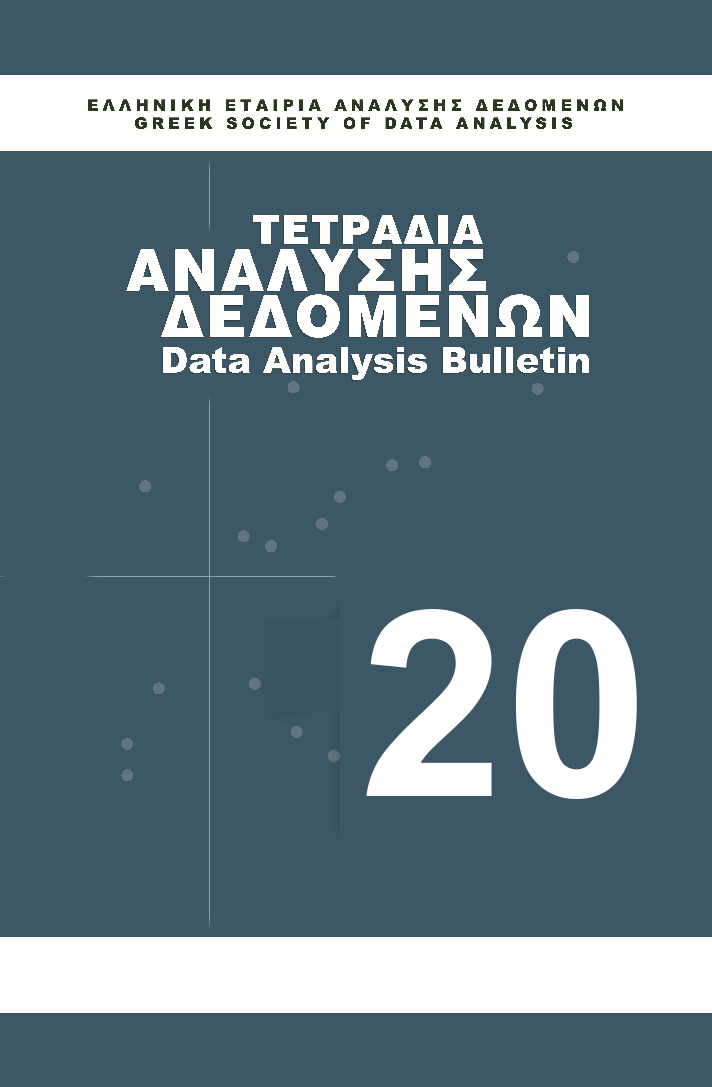
Abstract
In this study, the possibility of using certain methods of Data Analysis as a preparatory stage of Machine Learning methods to improve their predictive ability, was investigated. Data Analysis methods examined were: Principal Component Analysis, Multiple Correspondence Analysis and Non-Linear - Categorical Principal Component Analysis with optimal scaling. Machine Learning methods examined were Support Vector Machine (SVM) and more specifically Support Vector Classifier (SVC), Stochastic Gradient Descent (SGDClassifier), Naïve Bayes (GaussianNB), K-Nearest Neighbor (KNN), Decision Tree Classifier, Random Forest Classifier and Multinomial Logistic Regression. Tests were performed using data collected in a nationwide survey. The total sample involved 42,593 teenagers, who were interviewed and answered more than 155 questions regarding their eating habits. Body Mass Index (BMI) was set as a dependent variable. BMI was measured and used in the analyses as a quantitative variable, but also as a qualitative one, where the values of the index were divided into classes, based on the recommendations of the World Health Organization. According to the tests results for this data set, the prediction is more secure when we use as dependent variable BMI as a qualitive ordinal variable with four classes. Disinging with a data analysis strategy contributes to saving time and also to the selection of the best prediction model, while dimensionality reduction, if it does not improve tre predictive ability of the modes, at least contributes to the “interpretability” of the results.
Article Details
- How to Cite
-
Papafilippou, N., Kyrana, Z., Pratsinakis, E., Markos, A., & Menexes, G. (2024). Using Data Analytics methods before using Machine Learning algorithms: prediction on mixed data. Data Analysis Bulletin, 20(1), 32–44. Retrieved from https://ejournals.epublishing.ekt.gr/index.php/dab/article/view/33723
- Section
- Empirical studies

This work is licensed under a Creative Commons Attribution-NonCommercial-NoDerivatives 4.0 International License.
Authors who publish their work in the journal DATA ANALYSIS BULLETIN agree to the following terms:
1. Authors will not be charged any submission, processing or publication fees for their work. These costs are covered by the Greek Society of Data Analysis.
2. The copyright of papers published in the journal DATA ANALYSIS BULLETIN is protected by the Creative Commons Attribution-NonCommercial-NoDerivatives 4.0 International license. The Authors retain the Copyright and grant the journal the right of first publication. This license allows third party licensees to use the work in any form for non-commercial purposes only. If third parties modify or adapt the content, they must license the modified material for noncommercial purposes only. If others modify or adapt the material, they must license the modified material under identical terms.
3. Provided that the terms of the licence concerning the reference to the original author and the original publication in the journal DATA ANALYSIS BULLETIN are maintained.
4. Authors may enter into separate and additional contracts and agreements for the non-exclusive distribution of the work as published in the DATA ANALYSIS BULLETIN journal (e.g., deposit in academic repositories), provided that the acknowledgement and citation of the first publication in the DATA ANALYSIS BULLETIN journal is acknowledged.
5. The DATA ANALYSIS BULLETIN journal allows and encourages authors to deposit their work in institutional (e.g. the repository of the National Documentation Centre) or thematic repositories, after publication in DATA ANALYSIS BULLETIN and under Open Access conditions, as determined by their research funders and/or the institutions with which they collaborate, as appropriate. When submitting their work, authors should provide information on the publication of the work in the journal and the sources of funding for their research. Lists of institutional and thematic repositories by country are available at http://opendoar.org/countrylist.php. Authors can deposit their work free of charge in the repository www.zenodo.org, which is supported by OpenAIRE (www.openaire.eu ), as part of the European Commission's policies to support Open Academic Research.