Null Hypothesis Significance Testing: procedure, misconceptions and some suggestions for good practices
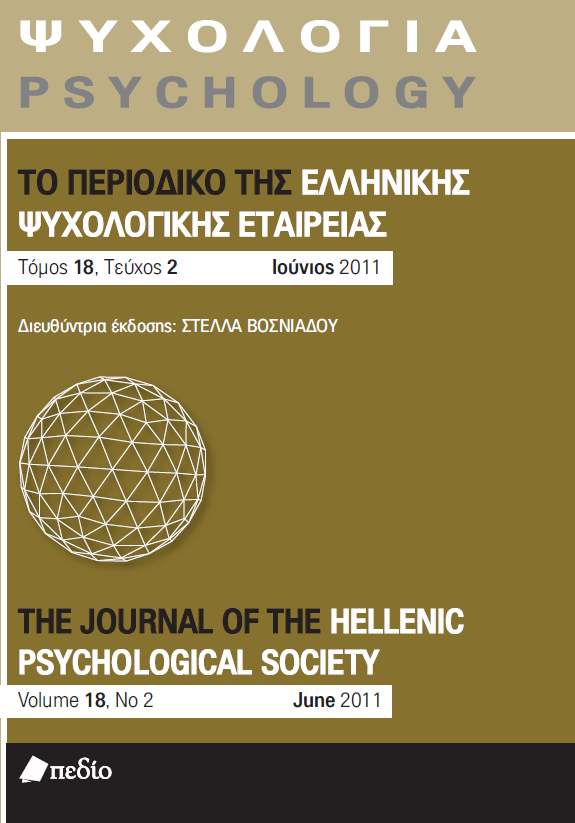
Abstract
The rationale of Null Hypothesis Significance Testing (NHST) is described, and the consequences of its hybridism are discussed. The paper presents examples published in “PSYCHOLOGY: The Journal of the HPS” refer to NHST and interpret its outcomes. We examined the 445 articles published between 1992 and 2010. We noted misuses of NHST and searched for any use of confidence intervals or error bars or use of these to support interpretation. Part of the paper focuses on the statistical-reform debate and provides detailed guidance about good statistical practices in the analysis of research data and the interpretation of findings. The proposed guide does not fall into the trap of mandating the use of particular procedures; it rather aims to support readers’ understanding of research results.
Article Details
- How to Cite
-
Ρούσσος Π. (2020). Null Hypothesis Significance Testing: procedure, misconceptions and some suggestions for good practices. Psychology: The Journal of the Hellenic Psychological Society, 18(2), 224–239. https://doi.org/10.12681/psy_hps.23720
- Issue
- Vol. 18 No. 2 (2011)
- Section
- BRIEF RESEARCH REPORTS

This work is licensed under a Creative Commons Attribution-ShareAlike 4.0 International License.
The journal PSYCHOLOGY adopts a Platinum open-access policy. Submission, processing or publication costs are waived by the Hellenic Psychological Society. Papers published in the journal PSYCHOLOGY are licensed under a 'Creative Commons Attribution-ShareAlike 4.0 International' licence. The authors reserve the copyright of their work and grant the journal the right of its first publication. Third-party licensees are allowed to use the published paper immediately after publication as they wish, provided they retain the defined by the license copyright formalities, regarding the reference to its author(s) and its initial publication in the journal PSYCHOLOGY. Moreover, any adjusted work should be shared under the same reuse rights, so with the same CC license.